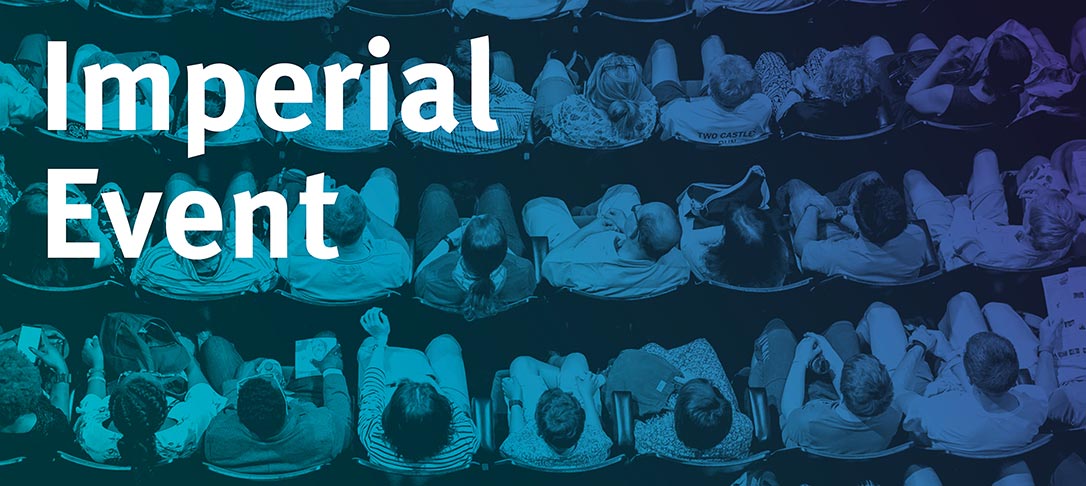
We study causal distributionally robust optimization (DRO) problems in a discrete-time dynamic setting where the model uncertainty is quantified using causal optimal transport. This discrepancy fully characterizes the spatial and temporal information of stochastic processes. Our approach focuses on two fundamental aspects: duality theory and sensitivity analysis. We establish a tractable dynamic duality for general penalized DRO. Additionally, we derive an explicit formula for the distributional sensitivity of value function to model uncertainty. We extend classical results to the so-called adapted objective functions. We illustrate our theoretical results with applications such as distributionally robust version of average value-at-risk, stochastic control, and optimal stopping.